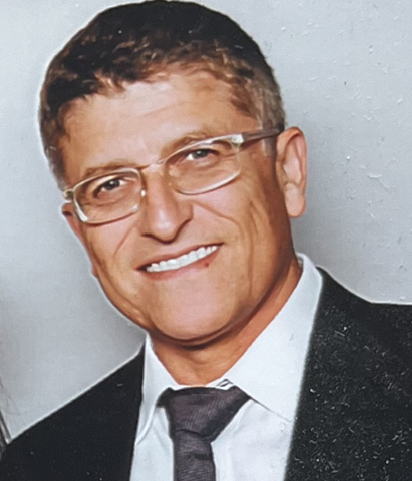
Daniel D. Gutierrez, Editor-in-Chief & Resident Data Scientist, insideAI News, is a practicing data scientist who’s been working with data long before the field came in vogue. He is especially excited about closely following the Generative AI revolution that’s taking place. As a technology journalist, he enjoys keeping a pulse on this fast-paced industry.
The landscape of artificial intelligence (AI) has rapidly evolved, with generative AI standing out as a transformative force across industries. For executives seeking to leverage cutting-edge technology to drive innovation and operational efficiency, understanding the core concepts of generative AI, such as transformers, multi-modal models, self-attention, and retrieval-augmented generation (RAG), is essential.
The Rise of Generative AI
Generative AI refers to systems capable of creating new content, such as text, images, music, and more, by learning from existing data. Unlike traditional AI, which often focuses on recognition and classification, generative AI emphasizes creativity and production. This ability opens a wealth of opportunities for businesses, from automating content creation to enhancing customer experiences and driving new product innovations.
Transformers: The Backbone of Modern AI
At the heart of many generative AI systems lies the transformer architecture. Introduced by Vaswani et al. in 2017, transformers have revolutionized the field of natural language processing (NLP). Their ability to process and generate human-like text with remarkable coherence has made them the backbone of popular AI models like OpenAI’s GPT and Google’s BERT.
Transformers operate using an encoder-decoder structure. The encoder processes input data and creates a representation, while the decoder generates output from this representation. This architecture enables the handling of long-range dependencies and complex patterns in data, which are crucial for generating meaningful and contextually accurate content.
Large Language Models: Scaling Up AI Capabilities
Building on the transformer architecture, Large Language Models (LLMs) have emerged as a powerful evolution in generative AI. LLMs, such as GPT-3 and GPT-4 from OpenAI, Claude 3.5 Sonnet from Anthropic, Gemini from Google, and Llama 3 from Meta (just to name a few of the most popular frontier models), are characterized by their immense scale, with billions of parameters that allow them to understand and generate text with unprecedented sophistication and nuance.
LLMs are trained on vast datasets, encompassing diverse text from books, articles, websites, and more. This extensive training enables them to generate human-like text, perform complex language tasks, and understand context with high accuracy. Their versatility makes LLMs suitable for a wide range of applications, from drafting emails and generating reports to coding and creating conversational agents.
For executives, LLMs offer several key advantages:
- Automation of Complex Tasks: LLMs can automate complex language tasks, freeing up human resources for more strategic activities.
- Improved Decision Support: By generating detailed reports and summaries, LLMs assist executives in making well-informed decisions.
- Enhanced Customer Interaction: LLM-powered chatbots and virtual assistants provide personalized customer service, improving user satisfaction.
Self-Attention: The Key to Understanding Context
A pivotal innovation within the transformer architecture is the self-attention mechanism. Self-attention allows the model to weigh the importance of different words in a sentence relative to each other. This mechanism helps the model understand context more effectively, as it can focus on relevant parts of the input when generating or interpreting text.
For example, in the sentence “The cat sat on the mat,” self-attention helps the model recognize that “cat” and “sat” are closely related, and “on the mat” provides context to the action. This understanding is crucial for generating coherent and contextually appropriate responses in conversational AI applications.
Multi-Modal Models: Bridging the Gap Between Modalities
While transformers have excelled in NLP, the integration of multi-modal models has pushed the boundaries of generative AI even further. Multi-modal models can process and generate content across different data types, such as text, images, and audio. This capability is instrumental for applications that require a holistic understanding of diverse data sources.
For instance, consider an AI system designed to create marketing campaigns. A multi-modal model can analyze market trends (text), customer demographics (data tables), and product images (visuals) to generate comprehensive and compelling marketing content. This integration of multiple data modalities enables businesses to harness the full spectrum of information at their disposal.
Retrieval-Augmented Generation (RAG): Enhancing Knowledge Integration
Retrieval-augmented generation (RAG) represents a significant advancement in generative AI by combining the strengths of retrieval-based and generation-based models. Traditional generative models rely solely on the data they were trained on, which can limit their ability to provide accurate and up-to-date information. RAG addresses this limitation by integrating an external retrieval mechanism.
RAG models can access a vast repository of external knowledge, such as databases, documents, or web pages, in real-time. When generating content, the model retrieves relevant information and incorporates it into the output. This approach ensures that the generated content is both contextually accurate and enriched with current knowledge.
For executives, RAG presents a powerful tool for applications like customer support, where AI can provide real-time, accurate responses by accessing the latest information. It also enhances research and development processes by facilitating the generation of reports and analyses that are informed by the most recent data and trends.
Implications for Business Leaders
Understanding and leveraging these advanced AI concepts can provide executives with a competitive edge in several ways:
- Enhanced Decision-Making: Generative AI can analyze vast amounts of data to generate insights and predictions, aiding executives in making informed decisions.
- Operational Efficiency: Automation of routine tasks, such as content creation, data analysis, and customer support, can free up valuable human resources and streamline operations.
- Innovation and Creativity: By harnessing the creative capabilities of generative AI, businesses can explore new product designs, marketing strategies, and customer engagement methods.
- Personalized Customer Experiences: Generative AI can create highly personalized content, from marketing materials to product recommendations, enhancing customer satisfaction and loyalty.
Conclusion
As generative AI continues to evolve, its potential applications across industries are boundless. For executives, understanding the foundational concepts of transformers, self-attention, multi-modal models, and retrieval-augmented generation is crucial. Embracing these technologies can drive innovation, enhance operational efficiency, and create new avenues for growth. By staying ahead of the curve, business leaders can harness the transformative power of generative AI to shape the future of their organizations.
Sign up for the free insideAI News newsletter.
Join us on Twitter: https://twitter.com/InsideBigData1
Join us on LinkedIn: https://www.linkedin.com/company/insideainews/
Join us on Facebook: https://www.facebook.com/insideAINEWSNOW