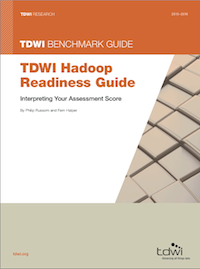
A recent TDWI Hadoop survey asked: “In your perception, what would be the most useful applications of Hadoop if your organization were to implement it?”3 Data warehousing (DW) and business intelligence (BI) use cases were by far the most common responses to the question. This is no surprise because DW/BI uses cases for Hadoop are well established. However, the prominence of non-DW/BI applications in the survey (e.g., archiving, content management, and operational applications) shows that these are emerging and will become more common. TDWI believes this is a sign that Hadoop usage is diversifying broadly across and within mainstream enterprises.
Among TDWI members, Hadoop regularly appears as a complementary extension of a data warehouse when warehouse data that doesn’t necessarily require the warehouse
is migrated to Hadoop. A similar extension is where data staging and data landing functions are migrated to Hadoop. “Fork-lifting” operational data stores to Hadoop is a trend that TDWI has just started seeing.
Some of the hottest BI user practices of recent years involve data exploration and discovery, which are critical to learning new facts about a business, as well as getting to know new big data and its potential business value. To enable the broadest possible exploration, some users
are collocating numerous large data sets on Hadoop. Data exploration is usually the first step in an analytic project, so it’s a fortuitous coincidence that Hadoop is also a capable computational platform and sandbox for advanced analytics. The trend with analytics on Hadoop is toward advanced forms of analytics, such as those based on machine learning, text analytics, graph, statistical analyses, and real-time analytics or event processing.
Data lakes and enterprise data hubs are two of the fastest-growing practices on Hadoop today. Both involve loading multiple massive data sets into Hadoop (easily reaching petabyte scale) with little or no preparation of the data. That way data ingestion is fast, simple, and cheap. To make up for minimal a priori data preparation, both lakes and hubs usually rely on post- storage data prep and data federation or virtualization techniques to model and transform data on the fly, on an as-needed basis. This gives analytics the agile ability to repurpose data (at analysis runtime) for open-ended exploration, discovery, analysis, and visualization.
For legal, audit, and compliance reasons, many corporations and other organizations are modernizing their enterprise data archiving facilities. Users are finding that Hadoop has favorable economics and scalability for modern active archives, whether involving non-traditional data (Web, machine, sensor, social) or traditional enterprise data.
Use cases for Hadoop with content, document, and records management (plus similar practices, such as e-mail archiving) are just now emerging.
Despite the established use cases just described, the current state of TDWI Hadoop has weaknesses or omissions that make its use challenging. For example, Hadoop is not a database management system (DBMS), so it lacks DBMS functions for schema and metadata management, indexing, transaction processing, ANSI SQL, granular security, and so on. Luckily, TDWI Hadoop gets better almost daily, and vendor products can compensate for many of these challenges.
All information that you supply is protected by our privacy policy. By submitting your information you agree to our Terms of Use.
* All fields required.