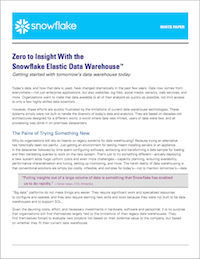
Today’s data, and how that data is used, have changed dramatically in the past few years. Data now comes from everywhere—not just enterprise applications, but also websites, log files, social media, sensors, web services, and more. Organizations want to make that data available to all of their analysts as quickly as possible, not limit access to only a few highly-skilled data scientists.
However, these efforts are quickly frustrated by the limitations of current data warehouse technologies. These systems simply were not built to handle the diversity of today’s data and analytics. They are based on decades-old architectures designed for a different world, a world where data was limited, users of data were few, and all processing was done in on-premises datacenters.
Why do organizations still rely so heavily on legacy systems for data warehousing? Because trying an alternative
has historically been too painful. Just getting an environment for testing meant installing servers or an appliance
in the datacenter followed by time spent configuring software, extracting and transforming a data sample for loading and then translating queries to work on the new system. That’s just to try something different—actually deploying
a new system adds huge upfront costs and even more challenges—capacity planning, ensuring availability, performance characterization and tuning, setting up monitoring, and more. The harsh reality of data warehousing is that conventional solutions are simply too costly, inflexible, and complex for today’s—not to mention tomorrow’s—data.
“Big data” platforms do not make things any easier. They require significant work and specialized resources
to configure and operate, and they also require learning new skills and tools because they were not built to be data warehouses and to support SQL.
Given the daunting costs, effort, and necessary investments in hardware, software and personnel, it is no surprise that organizations still find themselves largely tied to the limitations of their legacy data warehouses. They
find themselves forced to evaluate new projects not based on their potential value to the company, but based on whether they fit their current data warehouse.
All information that you supply is protected by our privacy policy. By submitting your information you agree to our Terms of Use.
* All fields required.