Supercharging AI with a Comprehensive Data Catalog and Robust Access Controls
As data grows in volume, AI becomes increasingly vital for analytical tasks within organizations. However, for AI to provide reliable and meaningful insights, it must be built with a comprehensive understanding of this data.
In addition, effective data access controls must be deployed to ensure that data remains accessible yet secure. These components ensure a strong foundation for AI tools that will greatly augment an organization’s analytical capabilities while simultaneously ensuring the responsible use of AI.
Know Your Data
There are many ways that AI can be utilized to address an organization’s needs. One powerful application is how AI-powered access-controlled data catalogs can enable businesses to generate reports without requiring deep technical knowledge. These reports are context-aware, accurate, and designed to meet specific access levels. AI can also be utilized to recommend the best datasets for specific projects based on access constraints, addressing project needs while ensuring compliance to security guidelines. Another application lies in AI’s ability to analyze ETL code, which can provide clear lineage tracking for data quality assessments by offering insights into data transformations, origins, and flow.
However, for these tools to be effective, they require a detailed understanding of the data they operate on. A comprehensive data catalog includes not only the raw data but also metadata, data lineage, and annotations from subject matter experts. Metadata—such as column names, data types, and measurement units—enables AI tools to interpret and analyze data accurately. Data lineage provides information on the origin of each dataset, any transformations applied, and integrations with other datasets, offering valuable context beyond metadata alone. Tracking data lineage through complex ETL (Extract, Transform, Load) processes is essential to provide this layer of transparency, but can be challenging to provide. Finally, expert notes and annotations contribute additional insights that help AI understand the data from a domain-specific perspective. Alongside the catalog, data access controls ensure that AI tools can operate within secure and compliant boundaries, allowing contextual analysis while safeguarding data privacy.
We’ll provide an example of these components by analyzing a data catalog of healthcare records. In this scenario, metadata might describe patient demographics and medical history data types, enabling AI to interpret each field correctly. Data lineage traces the data’s journey from clinical records to analytical dashboards, preserving essential context about each transformation. Expert annotations, such as clinician insights or diagnostic notes, enrich this context, helping AI distinguish between similar medical terms or conditions. Finally, access controls restrict the data and use of corresponding AI tools to authorized users, ensuring data privacy and regulatory compliance. This integrated approach improves the accuracy and reliability of AI-driven insights in a sensitive field.
Build an Effective Data Catalog with Access Controls
To build a data catalog that supports effective AI use while maintaining strict security, it’s essential to follow a structured approach that enriches data, tracks its origins, integrates expert insights, and controls access. The following steps outline the recommended practices to achieve a robust and reliable data catalog:
1. Metadata Enrichment: Ensure each dataset is equipped with complete metadata, including data types, units, and descriptions. Enrich metadata with standardized tags and detailed descriptions to improve AI’s interpretability and facilitate data discovery across the catalog.
2. Lineage Documentation: Maintain precise data lineage to track the origin, transformations, and interactions of datasets. Advanced AI-driven agents can analyze ETL scripts directly to trace lineage through each step and ensure the reliability of the data. For an in-depth discussion on this topic, refer to our previous blog post on using AI to track lineage in ETL pipelines.
3. Expert Annotations: Integrate annotations from subject matter experts to add contextual insights that enrich datasets. Choose tools that support collaborative data cataloging, allowing experts to contribute knowledge directly within the catalog. Annotation capabilities provide AI with domain-specific context, increasing the relevance and reliability of analyses.
4. Access Control Mechanisms: Implement precise access permissions to ensure data availability only to authorized users. Fine-tuned access settings ensure that sensitive data is accessible only to those with appropriate permissions, minimizing risk while supporting data governance.
Using these techniques to enhance data cataloging and control access strengthens data governance, ensuring the catalog is both secure and optimized for effective AI use.
Conclusion
A comprehensive data catalog with robust access control, complemented by expert insights, is essential for secure and effective AI-driven data management. By prioritizing these elements, organizations can empower AI systems to generate precise insights, automate reporting, and recommend data confidently.
About the Author
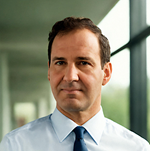
John Mark Suhy is CTO of Greystones Group. Mr. Suhy brings more than 20 years of enterprise architecture and software development experience with leading agencies including FBI, Sandia Labs, Department of State, US Treasury and the Intel community. Mr. Suhy authored the Government Edition of Neo4j, the world’s leading graph database supporting Artificial Intelligence/Machine Learning and Natural Language Processing. He also is the co-founder of the open source ONgDB and DozerDb graph database projects. Mr. Suhy is a frequent speaker at prestigious events such as RSA. He holds a B.S. in Computer Science from George Mason University in Virginia.
Sign up for the free insideAI News newsletter.
Join us on Twitter: https://twitter.com/InsideBigData1
Join us on LinkedIn: https://www.linkedin.com/company/insideainews/
Join us on Facebook: https://www.facebook.com/insideAINEWSNOW
Check us out on YouTube!