In a few short years, AI has transformed industries in ways few could have imagined, with large language models (LLMs) driving innovations in content creation, customer service, and beyond. These models, typified by applications such as ChatGPT, have made AI more accessible and demonstrated its potential to augment human capabilities. However, as impressive as these LLMs are, they represent only the beginning of AI’s potential. A more profound evolution is now emerging: Large Quantitative Models (LQMs), designed to tackle complex real-world problems in areas such as healthcare, climate science, and materials design, are set to revolutionize industries and unlock new AI-powered breakthroughs for some of the world’s greatest challenges.
From LLMs to LQMs: An Evolution in AI
LLMs have achieved remarkable success by making AI user-friendly and widely accessible. They excel at tasks like generating natural language, translating text, and providing customer support. For example, virtual assistants including Siri and Google Assistant are powered by LLMs that can process vast amounts of text data to answer questions and perform tasks in real time. LLMs are primarily trained on historical data, such as publicly available data on the internet, making them ideal for language-based tasks. While beneficial for content generation, LLMs are not designed to solve the physical, material, and scientific challenges facing the world today.
LQMs represent a shift toward physics-driven AI. Grounded in the known laws of nature and building on advanced computational simulations, LQMs can predict how complex systems—such as molecules, materials, and chemical reactions—interact in the physical world. In that way they transcend historical data by creating new, synthetic, highly reliable data based on first-principles physics. But, as AI models, LQMs can go a step further than simulations by also learning from that data to enable breakthroughs in industries like healthcare, energy, and materials science.
Revolutionizing Healthcare with LQMs
One of the most promising applications of LQMs is in healthcare, where they are transforming drug discovery. Traditional drug development is notoriously slow and expensive, often taking between 10 to 15 years to bring a new drug to market, with costs ranging from $1.1 billion to $4 billion. Moreover, the process is fraught with uncertainty: roughly 88% of drug candidates fail in clinical trials. This inefficiency leads to significant delays in addressing global health crises and unmet medical needs, particularly for rare diseases and complex conditions like neurodegenerative disorders.
Through rapid in silico simulations, LQMs test billions of potential molecular interactions, reducing the need for costly and time-consuming experiments in early-stage research. The LQMs can also go beyond pure physics-based simulation by combining this molecular data with our knowledge of biology, leading to predictions of a drug’s efficacy and potential toxicities, significantly de-risking human trials. By optimizing drug candidates early in the process, LQMs reduce the likelihood of failure in clinical trials, saving valuable resources and accelerating the time to market for life-saving therapies. Crucially, neither a pure first-principles simulation approach nor a traditional physics-agnostic AI approach is able to tackle these challenges. LQMs combining strengths of both are able to make progress.
LQMs hold particular promise in tackling the world’s most challenging diseases, from cancers to Alzheimer’s and Parkinson’s. For example, at the University of California, San Francisco’s Institute for Neurodegenerative Diseases, researchers have achieved a 50x to 100x increase in hit rates for positive interactions between drug compounds and biological targets using LQMs—far surpassing what was possible with earlier computational tools. This breakthrough has generated promising new compounds for Alzheimer’s disease and other conditions that would have otherwise taken decades to develop.
By enabling faster and more cost-effective drug development, these models are accelerating the discovery of new treatments, helping address unmet medical needs, and lowering the cost burden on healthcare systems worldwide.
Addressing the Climate Crisis with LQMs
The climate crisis presents another urgent challenge for governments and industries alike. LQMs are emerging as a powerful driver of climate innovation across sectors like energy storage and materials science.
Energy storage is a key component of the transition to renewable energy sources like solar and wind. As the world shifts toward electric vehicles (EVs) and renewable power grids, advanced battery technologies are needed to store and distribute clean energy efficiently. However, developing these next-generation battery chemistries has traditionally been a long and resource-intensive process, often requiring years of testing.
LQMs are reshaping this field by enabling researchers to optimize battery chemistries and accelerate the development of innovative battery designs. For example, leading battery technology company NOVONIX leverages LQMs to streamline cycle-life testing for advanced batteries. Using synthetic data generated by quantitative AI-powered simulations, NOVONIX can now predict the life cycle of new battery designs 20 times faster than traditional lab testing. By using data from just the first 50 cycles to predict performance at 1,000 cycles, LQMs help NOVONIX focus R&D efforts on the most promising designs and chemistries. These insights enable faster market deployment of new batteries, supporting the growing demand for electric vehicles, renewable energy storage, and electrification in the building sector.
Sustainable materials design is another area where LQMs are making a difference. The global construction industry is a major contributor to CO2 emissions, responsible for approximately 21% of global CO2 output.
LQMs are also revolutionizing sustainable materials design, particularly in the construction industry, which accounts for 21% of global CO2 emissions. With global building demand expected to rise by 35% by 2030, LQMs are being used to create lighter, stronger, and greener materials that reduce environmental impact and energy consumption. For example, cement production, responsible for 8% of global CO2 emissions, relies heavily on calcium extracted from limestone, a process that requires high heat from fossil fuels. LQM-powered simulations are now helping researchers identify alternative binding agents or find ways of strengthening concrete, reducing both concrete consumption and its carbon footprint.
Creating new materials can also impact other sectors such as transportation. Developing lighter, stronger, more durable materials with improved strength-to-weight ratio could make automobiles and airplanes more fuel-efficient while retaining or improving their current safety standards.
The Path Forward: Collaborative Innovation for Global Solutions
Over the past few years, LLMs from OpenAI, Meta, Anthropic, and others have transformed how AI and its applications are perceived across industries. Now, leveraging LQMs, we are charting a new frontier of scientific discovery and industrial innovation.
The economic potential of LQMs is vast. By enabling faster innovation cycles and reducing the cost of R&D, they can drive breakthroughs across sectors and help solve complex global challenges. This remarkable technology has potential to unlock economic growth, fueling job creation and enhancing productivity in industries worldwide. While powerful, LQMs are also complex, highly specialized, and resource-intensive. Along with their potential benefits, there is corresponding concern for widening of global gaps in the digital economy.
As with other frontier technologies, quantitative AI’s potential cannot be fully realized without collaboration between the public and private sectors and among nation-states. Governments, research institutions, and industries must work together across borders to invest in the infrastructure, computational resources, and regulatory frameworks required to develop LQM-driven solutions at scale.
Public-private partnerships, such as those seen in the Clean Energy Ministerial and other global initiatives, will be fundamental in deploying LQMs to address global challenges spanning climate change, public health, and economic sustainability. By fostering cross-sector collaboration and investing in LQM development, governments and industry leaders can harness their full potential to address the most profound issues of our time.
About the Author
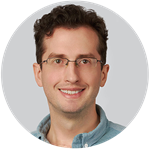
Dr. Stefan Leichenauer, a trailblazer in AI and Quantum technologies, serves as the Vice President of Engineering and lead scientist at SandboxAQ. As the inaugural member of the Sandbox team at Alphabet, Stefan played a pivotal role in transforming theoretical concepts into tangible real-world applications. His visionary approach and technical acumen led to the initiation of groundbreaking projects, shaping the foundation of SandboxAQ solutions. Stefan received his Ph.D. in Physics from UC Berkeley, and has held positions in Physics at UC Berkeley and Caltech as a leading researcher.
Sign up for the free insideAI News newsletter.
Join us on Twitter: https://twitter.com/InsideBigData1
Join us on LinkedIn: https://www.linkedin.com/company/insideainews/
Join us on Facebook: https://www.facebook.com/insideAINEWSNOW
Check us out on YouTube!