But those who figure out how to overcome hurdles and demonstrate endurance can win big
After years of hearing about the data deluge, it turns out we actually need more. Why? Because AI models are hungry, and they’re devouring data at an incredible rate.
ChatGPT was trained on 300 billion words. To put that in perspective, reading a novel a day for 80 years would only account for about 3 billion words – that’s less than 1% of what was used to train ChatGPT. But even 300 billion words is just a drop in the bucket. Databricks’ DBRX, the last model trained before GPT 4.0, consumed 12 TRILLION data points. Research suggests the growing demand for AI training data could outpace the total stock of public human text data as early as 2026 – even slightly earlier if models are overtrained.
Model overtraining occurs when AI models are developed using AI-generated data. This snake-eating-its-tail issue can result in a narrower range of outputs, among other issues. AI trained repeatedly on AI-generated text may just generate long lists or repeat itself. A model trained repeatedly on AI-generated images, for example, would eventually make all faces look similar.
With all of the AI-generated data floating around, the risk of AI overtraining is growing. If AI grabs data off the internet, you may unknowingly use AI-generated data and introduce bias.
That’s not to say that training AI models on AI-generated data is an entirely bad thing.
Synthetic data can be quite useful. In training autonomous vehicles, such data allows models to simulate every known driving scenario so that vehicles can function properly. AI-generated data is also valuable in life sciences. Imagine there’s a rare disease, but because it’s so rare, there’s not enough existing information to build AI models to detect or treat it. You could build an AI model with simulated data of that disease and use real data to validate the training.
However, there’s another problem with synthetic data. It makes the GPU do double duty. First, the GPU has to create the data. Then it uses that generated data to train. GPUs can scale to do the extra work, but they’re extremely computing- and energy-intensive, so it comes at a cost.
That’s a lot to process – both from a data standpoint and just to get your head around the other potential consequences. There is tremendous value in AI, but evolving challenges and the reality that about 90% of AI proof-of-concept pilots won’t move into production in the near future are creating AI fatigue. With this headlong rush into AI and finding where it can add value, the expectation was that this would be a sprint not a marathon.
Winning with AI will take endurance. While only 5%-10% of the AI use cases organizations are experimenting with now could bring opportunities, as Everest Group recently explained, those AI implementations “could have a huge impact on their company and are worth pursuing.”
Here are a few ways that you can stretch and hydrate as you condition yourself for the long run.
Lean into small language models
Large language models (LLMs) aren’t the only option. Small language models (SLMs) are useful, too. An SLM is the result of a highly refined LLM. Through refinement and concentrated information, organizations can create SLMs to be relevant to exact use cases.
SLMs are a good choice when you have very specific outcomes and intended designs in mind.
Say you want a train control system to interpret what a train is doing while it’s going down the track. The wide net cast by the open-source LLM’s is not entirely relevant, out of the box. Instead, these models should be concentrated to only the very specific requirements of the outcome. For the train control system, these models should be reduced and refined with the operating guides and technical documents that best understand what is going on.
Now, that’s great for the train operator, but if I’m teaching my daughter about monarch butterflies with ChatGPT, the likelihood of finding an SLM dedicated to just butterflies is low. Instead, we can benefit from the general knowledge that LLMs provide.
Your choice will depend on your organization and how much time and energy you invest into AI. While SLMs still require a tremendous amount of focused data to be effective, small language models tend to be more efficient. They require less data, are less costly and can be more efficient to operate. These considerations will be increasingly important as companies scale from one AI model to potentially thousands of AI models and use cases.
Adopt modern data infrastructure
GPUs that power our AI outcomes are continuing to evolve exponentially, consuming whatever data and powering whatever use-cases we throw at them. This progress will be at the cost of our ESG goals, impacting key sustainability initiatives. Like my children, they are power-hungry monsters.
However, if you can improve the infrastructure on the periphery of the GPU, you can indirectly realize better sustainability, economics and density. Here are some ways to do that:
- Adopt tools to cleanse and label data.
- Seek suppliers that are committed to sustainability.
- Embrace storage solutions with an ENERGY STAR rating.
- Engage with partners to continually improve performance and sustainability.
Go at it methodically, as a team
There’s no denying that this is difficult, but the value of AI success is huge.
Organizations must approach AI as a team. No single perspective will put you on the right track. To be successful, you need a perspective from the entire organization, ensuring that you are solving the right problem, the right way. This will also help avoid bias and prevent you from creating an ineffective solution. In some cases, Llama3 might be the right choice. In other cases, it might be preferable to go with a custom SLM. It depends on what you’re trying to do, and it’s definitely not one-size-fits-all.
Evaluate your organization. Define the results you want to go for. Then build your way there.
Winning with AI is not going to be easy. We’re running out of data, model overtraining is a reality and there will be other uphill battles. But we’re also seeing greater maturity in AI and the technologies that support it, retrieval augmentation (RAG) has become mainstream and is continuing to mature, a more efficient way to create SLMs will come into play and more companies are adopting modern data infrastructure.
Evolving developments are bringing greater scale, simplicity and sustainability to AI.
About the Author
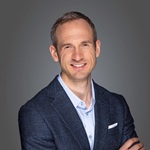
As Chief Technology Officer for Artificial Intelligence, Jason Hardy is responsible for the creation and curation of Hitachi Vantara’s AI strategy and portfolio. He is defining the future and strategic direction of Hitachi iQ, the company’s AI Platform, and cultivating a level of trust and credibility across the market by fostering strong working relationships with customers and partners, and leading public-facing events. Jason represents the company externally by communicating the company’s vision and value proposition for AI and by collaborating with key partners to develop comprehensive go-to-market strategies.
Sign up for the free insideAI News newsletter.
Join us on Twitter: https://twitter.com/InsideBigData1
Join us on LinkedIn: https://www.linkedin.com/company/insideainews/
Join us on Facebook: https://www.facebook.com/insideAINEWSNOW
Check us out on YouTube!