The topic of artificial intelligence (AI) has permeated nearly every boardroom around the world. And the discussion is no longer about whether to adopt AI, but how quickly it can be integrated into business operations. This urgency, while understandable, often overlooks a less glamorous yet critical preliminary requirement: robust data management that ensures the quality and integrity of the data powering these intelligent systems. This is the true foundation for successful, responsible AI integration.
The challenge of dark data
At the heart of this issue is “dark data”—unstructured, untagged and unused information silently accumulating within an organization’s digital infrastructure. Far from being a minor concern, on average, this data makes up more than 50% of a company’s total data volume. As businesses turn to large language models to drive generative AI and other AI-powered technologies, the implications of this dark data become both profound and potentially hazardous.
When used for machine learning by AI systems, this unmanaged data can lead to compromised decision-making, biased outputs and even legal repercussions. Imagine an AI model inadvertently trained on proprietary intellectual property or outdated and incomplete data. Perhaps even more concerning is the risk of unknown potentially sensitive personal information.
As AI adoption accelerates, so are regulations surrounding the usage and privacy of personal data. From the European Union’s General Data Protection Regulation to the multitude of state-level laws such as the California Consumer Privacy Act and industry-specific regulations like the Health Insurance Portability and Accountability Act, organizations face a complex web of data privacy compliance requirements. Unknowingly allowing AI systems to access potentially sensitive personal information could mean running afoul of these laws.
Responsible AI by managing dark data
To mitigate these risks and harness AI’s full potential, organizations must proactively manage their data. This approach begins with a comprehensive understanding of all data within the enterprise—not just the structured, easily categorized information but also the elusive log files, sensor data, draft documents and other types of dark data lurking in forgotten corners of their digital infrastructure. It also involves establishing clear data provenance and tracking the origins, movement and transformations of data throughout its lifecycle.
Implementing robust data monitoring, classification and analysis tools is crucial. These technologies can help organizations gain visibility into their entire data estates. By understanding what data they have and where it resides, organizations can make better informed decisions about how to use it in AI applications to ensure their AI models are trained on high-quality data sets.
It is important to pay extra special attention to identifying and classifying potentially sensitive personal information. A key best practice is to align an organization’s data governance policies with the most stringent compliance requirements that apply to the business. This approach ensures that current complaints needs are met and the organization is well positioned to adapt quickly as regulations evolve. By implementing comprehensive data management strategies that account for the strictest privacy and security standards, organizations can build a foundation of trust with customers and stakeholders while avoiding costly compliance violations.
In closing
As we enter this new era, the imperative is clear: organizations must prioritize understanding and managing their data as a prerequisite to integrating AI into their business processes. This means addressing the challenge of dark data and also fostering a culture of data awareness and responsibility throughout the organization.
Investing in data literacy programs for employees, establishing clear data governance policies and leveraging advanced data management technologies are all important steps in this journey. By taking these actions, businesses can unlock the full potential of their data, driving innovation through AI while maintaining quality and compliance.
About the Author
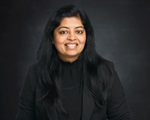
Soniya Bopache, vice president and general manager, data compliance and governance at Veritas Technologies. Soniya leads the vision, strategy, and delivery of the Veritas data compliance portfolio and has extensive experience in cloud migration, cloud deliveries, and managing SaaS-hosted offerings. Soniya earned a master’s degree in software engineering from the Birla Institute of Technology and Science in Pilani, India.
Sign up for the free insideAI News newsletter.
Join us on Twitter: https://twitter.com/InsideBigData1
Join us on LinkedIn: https://www.linkedin.com/company/insideainews/
Join us on Facebook: https://www.facebook.com/insideAINEWSNOW