The generative capabilities of foundation models are producing a profound effect on the financial services vertical. One of the most compelling applications of this form of advanced machine learning is the mitigation of financial crimes.
There’s a careful bifurcation of the impact of generative models for this important facet of financial services, the first of which benefits “our adversaries in the financial crimes space,” specified Stu Bradley, SAS Senior Vice President of Risk, Fraud and Compliance Solutions. Fraudsters have been relying on these machine learning capabilities to perpetuate a number of forms of fraud, including:
- Phishing: According to Bradley, “With the release of ChatGPT, you notice how much more real phishing attempts have become: whether emails, messages, or from text messages.”
- Deep Fakes: Deep fakes are an application of advanced neural networks frequently used to induce fraud. Typically, deep fakes entail rendering images.
- Audio Deep Fakes: However, deep fakes can also pertain to audio data, which oftentimes involve re-creating someone’s “voice to get in and around advanced authentication capabilities for multifactor authentication,” Bradley noted.
Nevertheless, generative machine learning models can be equally unremitting in their capabilities for checking financial crimes. When properly applied, they can streamline operational processes related to data collection, summarization, and link analysis, as well as create synthetic data of rare financial events.
The former directly assists investigators seeking to identify, minimize, and prevent criminal activity in this space. The latter immensely improves the predictive prowess of machine learning models trained to do many of the same things.
Synthetic Data
The synthetic data phenomenon actually predates the media maelstrom that arose in the wake of what many have termed Generative AI. With synthetic data, models generate additional data that is statistically similar to the characteristics of an existent dataset or data point.
“When looking at financial crimes and rare event detection, it’s hard to build models because the signal can be so limited,” Bradley divulged. “Being able to use synthetic data to generate signals, and slightly different signals representing changes into fraud patterns, are powerful tools so you can train and build models so they can be more agile.” Generative models can create synthetic data to broaden the amount of data for rare events involving crimes like money laundering, identity theft, and others.
Improved
Because these events are so rare in the host of transaction data a particular financial institution might have, it can be difficult to train machine learning models without synthetic data. Such models require training data to identify these events when they occur in the future. This scarcity of training data “can result in a lack of efficacy in the models,” Bradley commented. “But, because these events are rare, there’s also a limited understanding of how those fraud patterns and trends change over time, too.”
Synthetic data overcomes these barriers, generates a robust supply of labeled training data, and drastically improves the effectiveness of predictive models for thwarting financial crimes. “Financial institutions have examples of transactions with stolen credit card credentials,” Bradley explained. “Synthetic data generates additional data that replicates the appearance of stolen credentials. That information could train a model to identify when that happens in reality.”
Operational Effectiveness
Certain facets of the work financial crimes investigators do is extremely suitable for the generative prowess of foundation models. “The greatest adoption for Generative AI to date has been around driving effectiveness from an operational perspective,” Bradley revealed. “Think about the ability to address alerts from a fraud perspective and get more proactive.”
Instead of manually collating information pertaining to alerts, investigators can now use applications of generative models to collect all the relevant data pertaining to an alert, summarize the data, and pinpoint how the data links to additional accounts or activities. “If we can use Generative AI, Large Language Models, for example, to pull in data and information and summarize it so an investigator’s time can be used investigating and validating, [which is] what their intended job was meant to be, we’re going to have a much more operationally efficient program that allows them to more quickly respond,” Bradley indicated.
Co-Pilots, Virtual Assistants
Whether actuated in the form of virtual assistants or co-pilots, generative models can assist investigators by collecting various data about customers, accounts, and historic transactions. In addition to summarizing this information in relation to the alert, these models can also perform link analysis—which is vital for everything from stipulations like Know Your Customer to elaborate counterfeiting schemes.
“Investigators need to be able to understand any linkages to other accounts, either by transaction or non-transactional data, and all the different linkages from that account or customer to other accounts, or customers, part of an organized criminal ring,” Bradley mentioned. “That can be a very time consuming process.” Generative models can automate this process, expedite it, and enable investigators to complete their work at a greater scale and degree of efficiency.
Investigator in the Loop
Generative models are gradually reshaping the nature of financial crimes. They can help and hinder both investigators and the fraudsters perpetuating criminal activity. Applying synthetic data to increase the accuracy of machine learning models for counteracting fraud—and employing co-pilots powered by generative models to assist investigators in their work—has the tremendous potential to help the financial industry. That potential may be best realized when organizations monitor, govern, and manage the underlying models that are influencing how financial crimes are combated. That way, financial institutions are “not preventing people from accessing their funds or a social service,” Bradley cautioned. Temperance and human oversight of the outputs of initiatives involving generative models is therefore required to ensure their use proves salutary.
About the Author
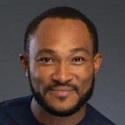
Jelani Harper is an editorial consultant servicing the information technology market. He specializes in data-driven applications focused on semantic technologies, data governance and analytics.
Sign up for the free insideAI News newsletter.
Join us on Twitter: https://twitter.com/InsideBigData1
Join us on LinkedIn: https://www.linkedin.com/company/insidebigdata/
Join us on Facebook: https://www.facebook.com/insideAI NewsNOW