For organizations everywhere—regardless of industry, size, or area of focus—data governance has reached a critical inflection point. It has long been established as a vital area of risk management predicated on achieving regulatory compliance, maintaining data privacy, and ensuring ongoing sustainability of data as an enterprise asset.
Today, however, it’s bounding beyond its risk management capabilities to boldly enter the arena of operations as one of the most viable constructs for either determining or influencing data-driven action. Moreover, recent developments have enabled it to do so dynamically, almost instantly, and potentially with as much sway as the downstream analytics and resulting decision-making that inevitably follows.
“One of the strong themes for Gartner is the idea of active metadata,” TopQuadrant CEO Irene Polikoff acknowledged. “One aspect of that is it’s directly actionable; it’s actually used in real time by operational systems to do various things.”
The operational functionality data governance will deliver in 2022 and beyond pertains to metadata management, data modeling, data stewardship, machine learning and Artificial intelligence, and various other components.
Its transition from a primarily static, passive set of principles and protocols to real-time applicability for a range of use cases producing business value naturally supports what is swiftly becoming a data economy in which firms are “moving towards a data marketplace,” added Purnima Kuchikulla, Privacera Director of Customer Success.
Operational Data Modeling
Some of the most meaningful operational action derived from data governance stems from data modeling. The interchange of data between varying systems as part of a collective data fabric is more indispensable than ever, with more organizations adopting this data management approach. Highly expressive data models with clear semantics and taxonomies can leverage machine intelligence to infer how the various schemas of different data systems can be blended for what TopQuadrant CTO Ralph Hodgson called frictionless integration. “You have similar information in different systems and the governance solution holds mappings between how it’s expressed in these different systems,” Polikoff explained. “You can involve the governance solution in real-time when you need to communicate between those systems.”
This approach pares costs, time, and effort for data integration by “avoiding the need for special programs to be written to take advantage of what’s done in the governance world,” Hodgson observed. More importantly, by using a system of constraints and smart inferences capability, organizations avail themselves of “the ability to generate new insights,” Hodgson pointed out. The most convincing example is for regulatory compliance, in which logical inferences about data access for one data source, regulation, or group of users can impact that for another source, regulation, or user group to automate compliance measures.
Metadata Insights
Inferences about metadata in data models can streamline taxonomies for media and entertainment industry content engines, for example, across global and local sources for near real-time results. Cognitive computing techniques can quickly automate the input of metadata; otherwise “metadata descriptions and their keywords would all be manual,” confirmed Contentserv CMO Jennifer Krizanek. In other examples, detailed visibility into metadata can either presage events or provide an infallible roadmap of previous ones to ensure staples of data lineage and data quality. Connecting metadata with what Datafold CEO Gleb Mezhanskiy termed a “metadata graph” delivers the following benefits at enterprise scale:
- Data Lineage and BI: The traceability provided by metadata is imperative for understanding—and trusting—the information in analytics. In this use case, “if there is a BI dashboard that someone looks at, it can reveal who looks at it and where does that data come from,” Mezhanskiy mentioned.
- Root Cause Analysis: Any outliers or aberrations in processes related to analytics are easily illustrated by analyzing metadata. “If someone sees an anomaly or something that looks like an error on a dashboard, because there’s a graph that shows how the data got there it’s easy to do root cause analysis,” Mezhanskiy maintained.
- Impact Analysis: By scrutinizing metadata about every facet of SQL used to generate information about tables, columns, and rows of data, “If someone makes a change to them you can tell exactly what’s going to happen because you can see it in the graph,” Mezhanskiy noted.
The capacity to operationalize metadata (particularly in graph environs) broadens the utility of this data governance facet to include everything from reference data to controlled vocabularies. Amassing metadata is critical for producing new insights from it—such as automatically propagating reference data rules throughout finance systems or those for records management in healthcare systems. Both machine learning and rules-based reasoning can create the resulting insights. “This is the other aspect of active metadata: not only what exists, but what can be generated or inferred from what’s explicitly stated,” Polikoff commented.
Activating Data Stewardship
The empowerment of data stewards is another direct repercussion of shifting data governance from passive to active employments. Modern innovations surrounding controlled data access that focus on data stewards are critical for speeding up the time required to use data while invariably conforming to governance standards about which users view what data. Shared data governance mechanisms issue “automatic approval and provision centralized governance rules in infrastructure like Snowflake,” Privacera CEO Balaji Ganesan revealed. “The sales data owner, for example, can say this is the portion of the data I’ll give access to John Doe.”
Automating the distribution of centralized governance policies into decentralized sources removes the IT bottleneck for data access, facilitates low latent data sharing, and positions data stewards—who know the data best—at the fore of delegating what data are accessed. It replaces “the friction in a process that used to take weeks,” Ganesan recalled, with a smooth one requiring minutes to share governed data at a pace equitable to that for operations. This method is predicated on active data stewardship, in which stewards “easily provision data and take back access if they need to automatically, but at the same time prove to a compliance person the full audit trail of who’s using the data and what for,” Ganesan indicated.
Data Quality
Data quality (as well as attendant aspects of data validation and data reliability) is the substratum upon which any form of data governance, particularly in operational settings, depends. “You can’t do automation or augment processes if your data’s not healthy or high quality to begin with,” Krizanek propounded. Embedding data governance staples like active metadata management into operational systems generating metadata in real-time requires data validation measures to ensure “it’s sensible and adheres to best practices,” Polikoff stipulated. There are several means of facilitating data quality at the levels in which it’s trustworthy for operations as well as conventional decision-making.
Some involve what Krizanek described as “traditional business rules engines, workflows, things that can automate that process and you have a dashboard that will tell you now it goes to this person, then this person needs to review and add this piece before it goes out the door.” There’s also a growing movement around what Mezhanskiy described as “data reliability engineering as a practice to get to better data quality and data reliability.” This practice is based on rigorously evaluating metadata so that “the data that’s correct represents reality,” Mezhanskiy denoted. Finally, Master Data Management solutions have transformations for “several data quality checks to validate data and normalize it,” Krizanek said.
Machine Learning
Another way data governance is extending its sphere to involve activities closer to operations is by gradually annexing different facets of AI. The use of intelligent inferences is the most eminent example; governance is also encompassing various aspects of machine learning. The features informing the ability for cognitive analytics models to learn can be treated as metadata. Dedicated feature stores for feature engineering are increasingly becoming influenced by governance mainstays of metadata management, data lineage, and more for a host of benefits.
According to Polikoff, “One of the trends is including machine learning model management and feature management into the data governance solution. The data governance expands to manage the machine learning model and the machine learning processes take advantage of the data governance solution because, it’s important to have models be accurate, to manage them, and understand the interrelationship of those models and where they’re being applied.”
Conversely, machine learning is regularly used to implement different facets of data governance by recommending actions pertaining to it. Krizanek articulated a use case in which such recommendations are used “to improve the quality of your data or the workflows it’s part of.”
Better Than Ever
The conception of active metadata is but one aspect of the overall metamorphosis of data governance from unchanging, historic-facing precepts about how data are used to the very means of using them in settings directly influencing production. Organizations can gain from this trend by effectively relying on operational data modeling, operational metadata management, and operational data stewardship. This approach is dependent on data quality, expands governance into data science, and is a progression that only behooves the organization making use of it.
About the Author
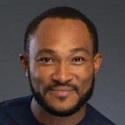
Jelani Harper is an editorial consultant servicing the information technology market. He specializes in data-driven applications focused on semantic technologies, data governance and analytics.
Sign up for the free insideAI News newsletter.
Join us on Twitter: @InsideBigData1 – https://twitter.com/InsideBigData1