
In this regular insideAI News feature we highlight our industry’s movers and shakers, companies that are pushing technology forward, and setting trends for innovation. We look at companies with a focus on big data, data science, machine learning, AI and deep learning – some new, some old, always leading, always dynamic. We also take deep dives into new technology promoted (or hyped) as “AI” or my favorite “AI-powered” to provide transparency for what’s really going on under the hood. Watch this column for intimate coverage of some pretty cool firms doing some pretty exciting things. Enjoy the ride!
In this installment of “AI Under the Hood” I introduce Kaskada, Inc., a Seattle-based early stage company founded in January 2018. Kaskada is a machine learning platform for feature engineering using event-based data.
As I started to learn more about this company earlier this year, my interest piqued due to their focus on “feature engineering,” a critical aspect of successful machine learning projects. Many Kagglers attribute success in various data challenges to “creative feature engineering.” Personally, I know the importance of feature engineering as I work on client projects, and I always stress its importance to my Introduction to Data Science students at UCLA. So any new techniques to help in this area, represent a potential winner in my eyes.
The startup recently announced it has raised $8 million in a series A round of funding, with participation from Voyager Capital, NextGen Venture Partners, Founders’ Co-op, and Walnut Street Capital Fund. This brings Kaskada’s total raised to $9.8 million, following a $1.8 million seed round in September 2018. The capital will be used to accelerate the company’s growth, expand its team of data engineers, and fulfill customer demand ahead of its flagship product’s launch in the first half of 2020.
Problem and Solution
Data scientists typically work in siloed tools that make standard development processes such as version control, code reviews and testing difficult. This means that their work cannon be used directly in customer-facing applications or business critical systems. Instead, engineering organizations must re-write machine learning features to connect data pipelines correctly and to make the feature vectors available to production systems. This work is repetitive and error prone. Worse, it typically takes weeks or even months to complete, slowing down experimentation and innovation in data teams.
Kaskada delivers an end-to-end platform for feature engineering and feature serving, including a collaborative interface for computing, storing, and serving features in production. Data scientists can own the end-to-end lifecycle for features, without needing help from engineering, and your users are automatically service accurate, up-to-date predictions based on their most recent behavior.
“My cofounder, Ben, and I had spent many years building distributed data processing systems at Google Cloud and had a first-hand view into how hard it can be to get to success with big data projects,” commented Davor Bonaci, CEO about the genesis of the company. “We left Google with the goal to make streaming and event-based data more accessible by focusing on solving real user problems. We wanted to take these great technologies and build a solution rather than a low-level framework. This led us on a journey that overturned widely-held beliefs about what users of data platforms really need to be successful. After conversations with countless companies, we found that productionizing and scaling machine learning is one of the biggest challenges facing data organizations across a wide range of industries, and ultimately decided to focus on building a machine learning platform.”
The following steps address the situation where designing features is an art, while deploying features is a pain:
- Connect to data – the Kaskada platform supports both historical and real-time streaming data sources for feature engineering. Data Scientists have access to all the data they need without requiring engineering assistance.
- Design and visualize features – data scientists use the Kaskada feature studio to explore data and design high quality features. Built-in visualization make it easy to understand feature distributions and clean and normalize feature values.
- Select and export features – data scientists choose relevant features to export and use to train their models. All features for your organization are shared and stored in a central “feature store” allowing collaboration across data science teams.
- Deploy to production – after the model are trained and ready, data scientists choose the final features to use in production. Data engineers simply call an API to get up-to-date feature vectors for each user.
“Kaskada helps organizations make better predictions and increase the speed of innovation by integrating data science and data engineering workflows,” added CEO Bonaci. “We deliver an end-to-end platform for feature engineering and feature serving, including a collaborative interface for data scientists and robust data infrastructure for computing, storing, and serving features in production.”
Conclusion
Kaskada is a machine learning company that enables collaboration among data scientists and data engineers. The company develops a machine learning studio for feature engineering using event-based data. Kaskada’s platform allows data scientists to unify the feature engineering process across their organizations with a single platform for feature creation and feature serving. If you’re a data scientist Kaskada solutions are definitely worth a close look.
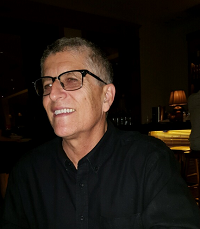
Contributed by Daniel D. Gutierrez, Managing Editor and Resident Data Scientist for insideAI News. In addition to being a tech journalist, Daniel also is a consultant in data scientist, author, educator and sits on a number of advisory boards for various start-up companies.
Sign up for the free insideAI News newsletter.